Which data is smart data?
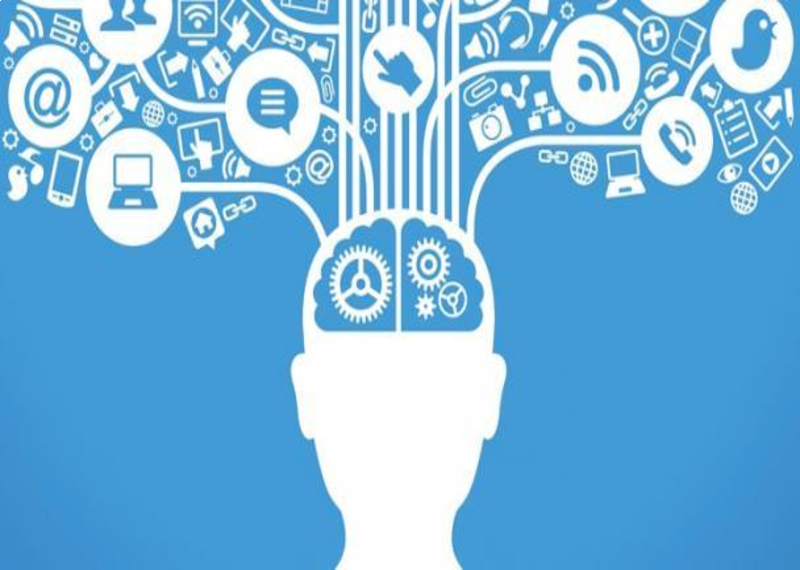
Successful data-driven businesses strive to improve data quality while reducing data organisation and preparation time. The goal is to create data that is ready for analysis quickly, and the best way to do this is to make data intelligent.
Successful data-driven businesses strive to improve data quality while reducing data organisation and preparation time. The goal is to create data that is ready for analysis quickly, and the best way to do this is to make data intelligent.
As we've seen, time is already the enemy of data-driven organizations, due to delays in collecting data and preparing it for traditional analysis. According to IDG Research's 2016 Data and Analytics Survey, 90 percent of respondents experienced pain points in areas such as data access, data transformation, data creation and collection, data migration, and data storage.
Further complicating the situation is the fact that data volumes are already huge and getting bigger, which is accelerating the speed of data flow through the organisation. While companies rely on vital intelligence from data to optimise the user experience, few can effectively manage to pull quality data out of the deluge at speed.
Getting full value from organisational data requires figuring out which bits are important on the fly, ensuring that the data quality is impeccable, and adding context that transforms the data into actionable information at the point of collection. If you can do that, you have smart data.
The difference between smart data and traditional data collection and analysis methods is profound, and impacts everything from improving customer experience and operational efficiency to reducing security threats.
How smart data helps
While definitions of smart data vary slightly, it is generally considered to be data that is prepared and organised at the point of collection to be ready for analysis at the highest possible quality and speed.
At a recent conference, Donna Roy, executive director of the U.S. Department of Homeland Security's Office of Information Sharing and Services, told FedTech that "her teams spend about 80% of their time just searching, inputting and preparing data for analysis". Roy believes that smart data will enable agencies to take the slack out of the process and work faster and smarter.
FedTech described Roy's description of smart data as "data that is independent of software, applications, devices or networks, but can still be used. It is data that describes and protects itself. It has its own context and semantics". This data is imbued with context, and this context is more closely associated with the data source.
"Smart data is information that actually makes sense," writes Wired in its article Big data, fast data, smart data. "It's the difference between looking at a long list of numbers indicating weekly sales and identifying peaks and troughs in sales volume. Algorithms turn meaningless numbers into actionable insights. Smart data is data from which signals and patterns are extracted by intelligent algorithms."
With traditional analytics, data is collected, nurtured and then processed on some fixed schedule, say daily or weekly. This workflow means that the results are often out of date by the time the data are taken into account. However, smart data is accessed at the point of collection and transformed for analysis, which helps to reduce delays in data preparation.
What does this mean in the business world? First and foremost, smart data helps companies to extract relevant data from the huge amount of data that is flooding in. Knowing what your data is telling you earlier is a huge boon in today's digital business world. Smart data can play a critical role in a range of activities, from healthcare monitoring and patient care to big data analytics, cloud migration, network and application performance management.
Consider, for example, the problem experienced by Bill Gillis, CIO at Beth Israel Deaconess Care Organization in Boston. His organization wanted to gain greater insight into patients' health through claims data, but this data is typically not available for analysis until 90 days after the event that caused the patient to contact the healthcare organization in the first place. This is clearly too long to respond meaningfully, rendering the data useless. If the data were available sooner, the organisation would suddenly have a wealth of new information that could be used to help patients.
Strategic tactics
The following considerations are important to develop a smart data strategy for your business:
Consider the data source. All data sources are not created equal, and it is important to find those that provide the most up-to-date and relevant data. For example, some network monitoring tools nowadays use unstructured machine data (log files, SNMP, etc.) that will at some point be indexed and archived for analysis. This approach has several limitations. Firstly, it results in a lot of data that needs to be sorted through, and secondly, it only collects data that can be logged, leaving potential blind spots. Two, the process produces old data. Using wired data for network visibility provides better intelligent data capture: a complete picture of what's happening, and if done right, it can be accessed, collected and transformed in real time.
Ensure data quality. By some estimates, bad data costs companies on average 12% of revenue. In fact, the old words garbage in, garbage out take on an exaggerated meaning given the critical use cases for smart data, from business analytics to operational roles in data security and application performance management. Building data quality requires a unified and consistent approach across the organisation, which is embedded in the corporate data governance manual.
Review the need for organisational change. Data analytics efforts tend to be centralized, but with smart data, value starts to accumulate soon after the initial assessment of the data, meaning there is more opportunity for action - and faster action - on data closer to the point of collection. This will impact both your technology strategies (are you equipped to exploit data faster?) and the way you assemble your teams to act on the data (will you need a more distributed structure to get the most out of the data?).
Embrace automation. Tools to automate data collection and transformation are vital, and the need will only grow as you try to extract value from the ever-increasing amount of data from ever more sources. There's simply no other way to get in front of the fire hose and expect to be able to meaningfully analyze, prioritize and interpret data.
As mentioned, however, there are different ways to approach the problem. Take, for example, intelligent data applications for network and application performance management, which require measurement of remote parts of the network for complete transparency. Hardware-based approaches may prove too costly and difficult to extend to cloud environments. This may not be an option for many organisations, but as part of the value of smart data lies in collecting it at the source, it is important to find an alternative. In this case, look for software tools that reduce costs while extending their reach to mixed environments, such as cloud and virtualised environments.
While it may be challenging, the ultimate success of your digital transformation efforts will depend on how good your data is and how quickly you can act on it. Smart data promises to help you make smarter decisions faster, and the companies that do this best will be the ones that come out on top.
How is smart data used? In the next two articles in this series, we'll show you some examples of how NETSCOUT smart data works.